This is a rather long blog post about how science is tackling brain complexity. If you are interested in the problem of complexity, or how scientists try to recreate brain biology in vitro, check out chapters 1 & 2. If you are curious about game-changing cell-electronic interfaces, go to chapter 3.
Background:
The scientific method has opened up our natural world to systematic human inquiry, allowing us to progressively gain a better understanding of ourselves and the universe we inhabit. Yet among the known unknowns and open mysteries, there is one question that even many scientists seem skeptical about if we can ever truly find an answer:
Will we ever be able to fully understand the complexities of the human brain?
Just from a medical perspective, understanding how the brain forms and how neurons, astrocytes, and other brain cells behave collectively is essential to finding the causes and treatment for developmental disorders, as well as understanding certain diseases.
Philosophically (and more dramatically), answering these fundamental questions of what makes us ‘us’ has even wider-reaching implications and might change our understanding of the human condition, if not the future of intelligent life in the universe. So the stakes can not be higher.
However, studying even basic mechanisms of brains or brain tissue in live animals or humans is invasive, methodologically challenging, and often not possible at all, given both ethical and technological limitations.
And this is just where the trouble starts.
Chapter 1: Charging against a complexity barrier
%2C-Nature%2C-2021).jpg)
Studying the brain can be frustrating
Researchers face a vast biological network made up of billions of neurons and trillions of synapses, forming information highways and processing units arranged in complicated three-dimensional (3D) microcircuitry. A diverse set of constantly interacting brain cells, from neurons and astrocytes to vasculature muscle and immune cells, act together to shape the network dynamics and computations ultimately expressing themselves as mental activities and behavior that make us human. The remarkable diversity of physiological, anatomical, and molecular mechanisms leading to the self-assembly of stereotyped scaffolds underlying brain networks are furthermore shaped and customized by the individual genomic building blocks at hand, on top of environmental factors, life experiences, and maybe even our choices.
There are layers upon layers of entangled intricacies, which can be neatly alliterated by the 4 C’s of brain complexity: Cells, Cytoarchitecture, Connectivity, and Change. Let’s have a quick look:
- Cell type diversity
The brain houses various specialized cell types commonly classified as belonging to either electrically excitable neurons or (non-excitable) “supportive” glial cells, but this traditional differentiation based on electrochemical signaling capacity is insufficiently precise to account for phenomena such as gliotransmission (Savtchouk I. and Volterra A, JNeurosci, 2018). In general, the boundaries of what defines a cell type are fluctuating in the times of emerging single-cell technologies. Several single-cell transcriptomic and epigenomic experiments suggest that we are vastly underestimating the number of specialized cell types any mammalian brain possesses (Yao, Z. et al., Nature, 2021).
It takes almost herculean efforts and manpower to create a representative cell atlas for just a single brain region, like the primary motor cortex. (BRAIN Initiative Cell Census Network (BICCN), Nature, 2021)
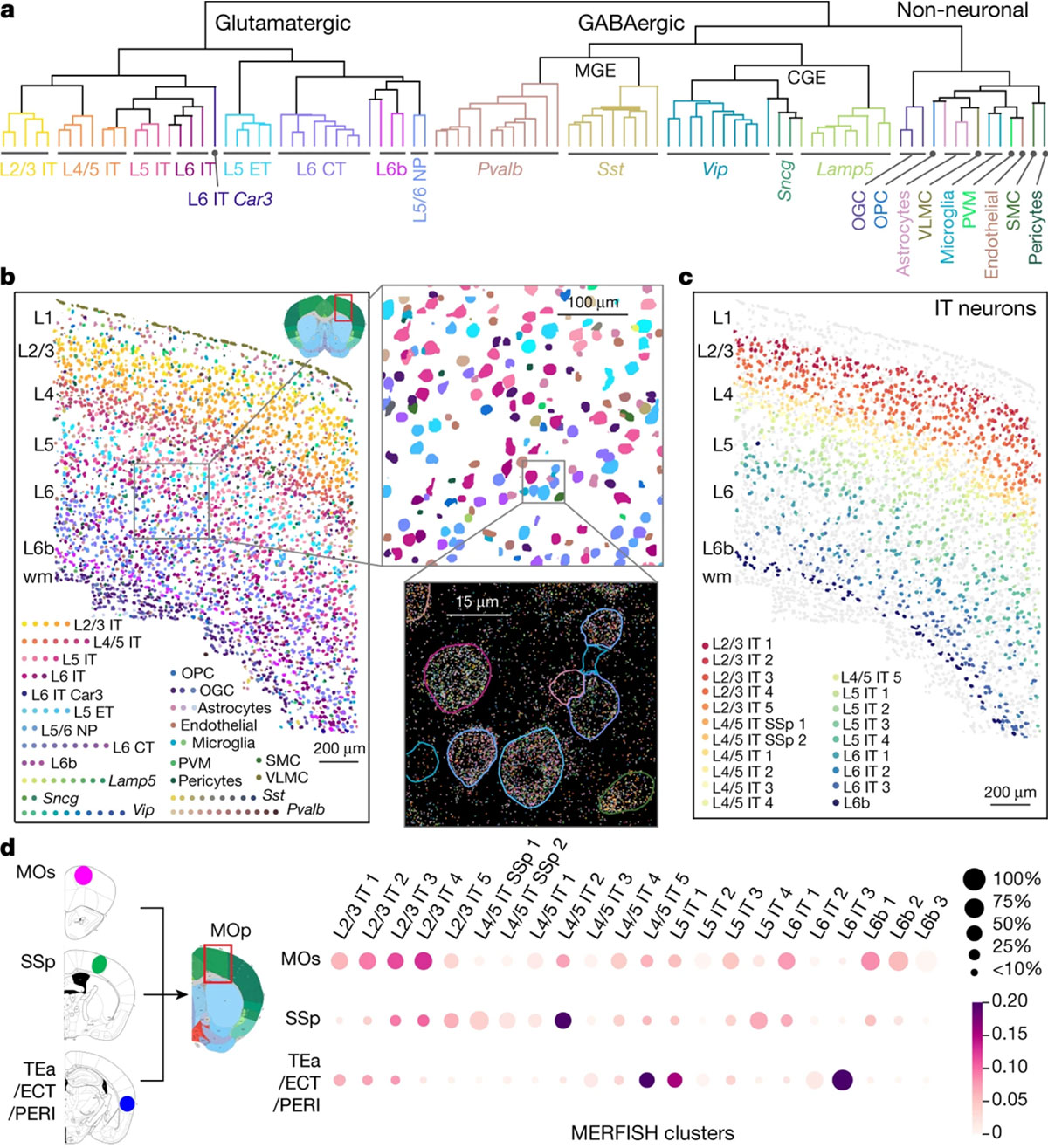
Cell atlases are essential to understanding the cellular diversity present in any given tissue, but figuring out which cell types are involved and in what quantities is just the beginning. One major limitation is the functional assessment of these single specialized cell types. Even cell-type-specific neuronal co-cultures can not recapitulate their true functions within a diverse network when outside their native and complex cytoarchitecture.
- Cytoarchitecture
Cytoarchitecture describes the 3D arrangement of cells within our brains. The most obvious way to study this architecture is through imaging. Yet even the best and most advanced large-scale structural and computational approaches (Amuts K. et al., Science, 2020) lack sufficient granularity to gain insights into computational or biological mechanisms.
Imagine a bird’s eye perspective, you sit in a plane and look down upon a big city. You might be able to differentiate between the harbor and downtown, maybe even in which regions are industry complexes and where people go for entertainment. If you’d really focus, you might even make out one or the other great building. But there is no chance that you will be able to tell what anyone human is doing, lest then keeping track of all of them at the same time. This is roughly where we stand now with current technologies. (but do check out the Human Brain Project’s interactive multilevel human brain atlas)
Another approach is anatomical, for example using serially stacked histological brain slices of post-mortem brains (Wojtasik M et al., Front. Neuroanat., 2020), helping with mapping large regions of the brain and their embedded cell types, but losing any chance of functional assessment.
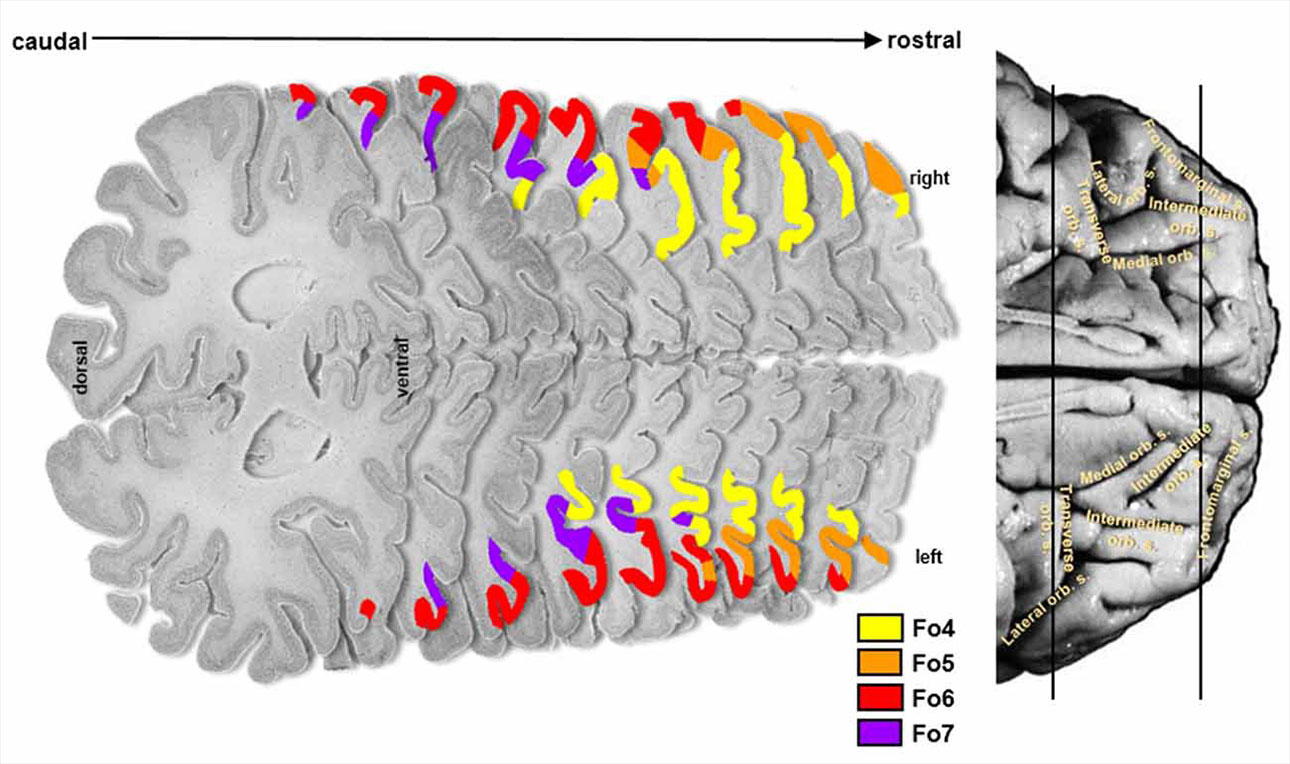
Scientists are well aware that they need to move beyond looking at the very large; getting a more detailed map of the structural organization of the cytoarchitecture it is an essential step towards understanding the development, function and diseases of the brain.
Beyond the cartographic aspect of this endeavor, they are necessary prerequisites to understand the organizational principles of the brain at its different spatial scales, including cellular and even subcellular components. Recent and classical data provide arguments supporting the hypothesis that cortical organization is composed of at least three different spatial scales — columns, micro-, and meso maps — thus emphasizing the multi-level nature of brain organization. Such cortical organization would directly influence the way that human brain models and simulation are conceptualized. — Katrin Amunts K and Zilles K, Neuron, 2015
- Connectivity
Synaptic connectivity between neurons allows for signal propagation and computation through the brain’s neuronal network, and the topology of those connections fundamentally shapes how our brain performs its functions. Since the 1950s, the mantra of ‘neurons that fire together, wire together’ advanced by psychologist Donald Hebb provided a compelling ‘assembly’ theory of how neurons from connections to each other, yet more advanced simulations in computational networks make clear that there is more to the story (Wu YK. and Zenke F., eLife, 2021).
What fires together, wires together.
Connectivity is an interesting problem to solve in brain research because network topology alone suggests there are multiple layers of functional organization, all acting at different scales ( Bullmore, E. and Sporns, O., Nat. Rev. Neurosci., 2009). To even just describe these complex interactions within their topologies, biology has shown to be insufficient and ill-equipped, that is why much of today’s connectivity research is based on math, statistics, and graph theory.
Convergent experimental and computational data suggest that there is interdependence in the organization of structural and functional networks. The topology, synchronizability and other dynamic properties of functional networks are strongly affected by small-world and other metrics of structural connectivity. Conversely, over a slower timescale the dynamics can modulate structural network topology. — Bullmore, E. and Sporns, O., Nat. Rev. Neurosci., 2009
In the past two decades, network neuroscience has helped to transform our understanding of the brain, with functional connectomics becoming a staple in neuroimaging studies and investigations of brain disorders (Fornita A. et al., Nat Rev Neurosci, 2015). However, functional connectomics studies currently also suffer from more basic problems around finding reliable and reproducible node definitions, edge construction, and network measurements, thus leading to large inner-individual variance and poor comparability between individuals (Jiang C. et al., bioarxiv, 2021).
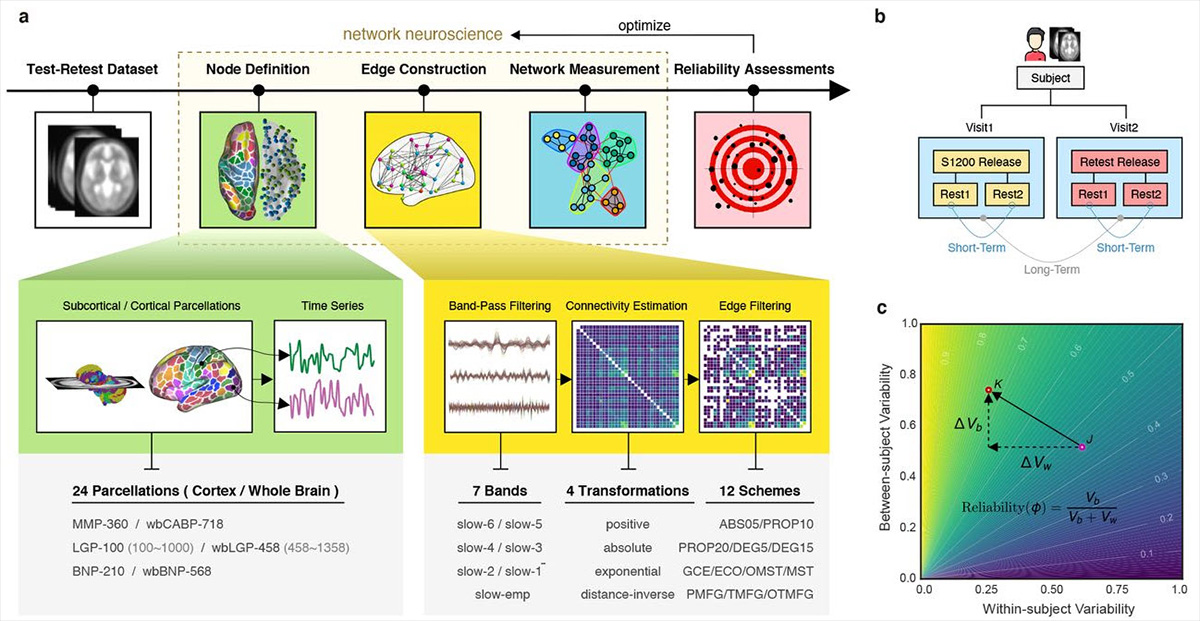
On top of variance, there is a more fundamental problem with network connectivity: It is flexible and changes throughout development, in response to experiences or damage, and over time.
This brings us to the last pillar of the brain’s complexity; dynamic change.
- Change (developmental and chaotic)
The brain is a flexible organ and neurons have the ability to modify the strength of their synaptic connections throughout our lifetime. Especially during development, disruptions like preterm birth cause pervasive deficiencies and delays in structural brain maturation that confer a higher risk for neurodevelopmental impairment and psychiatric illness in later life (Eyre M. et al., Brain, 2021).

More generally, neuroplasticity is an umbrella term that describes phenomena where the brain can reorganize itself and its neuronal connections in response to various sensory experiences and perturbations. Traditionally thought to predominantly occur during early development, neuroplasticity has since been shown to occur throughout a lifetime (Voss P. et al., Frontiers in Psychology, 2017) in response to many stimuli and circumstances, and with it, topological changes to the brain’s network organization.
Synaptic plasticity mechanisms and specific features of brain network share common principles that contribute to explain how neural plasticity influences brain network organization. Indeed, different forms of synaptic plasticity could be directly involved in generating specific brain networks’ characteristics. (Bassi MS. et al., Int. J. Mol. Sci., 2019)
Our brains’ tendency to change throughout our lifetime serves as a last and hard-to-tackle barrier of brain complexity:
Dynamic and developmental network self-organization means that the brain can not be studied as a stable system in equilibrium, but rather as a non-linear (i.e changing) complex system that continuously adapts to new experiences, circumstances, and perturbations in likely unique and chaotic fashion. And that is without even adding genetic diversity between individuals, personal life trajectory, and randomness to the list of influencing parameters.
How can we hope to ever study these intelligent and self-organizing biological networks if we cannot even (maybe ever) observe them at an appropriate scale?
Chapter 2: Human brain development, but in a dish
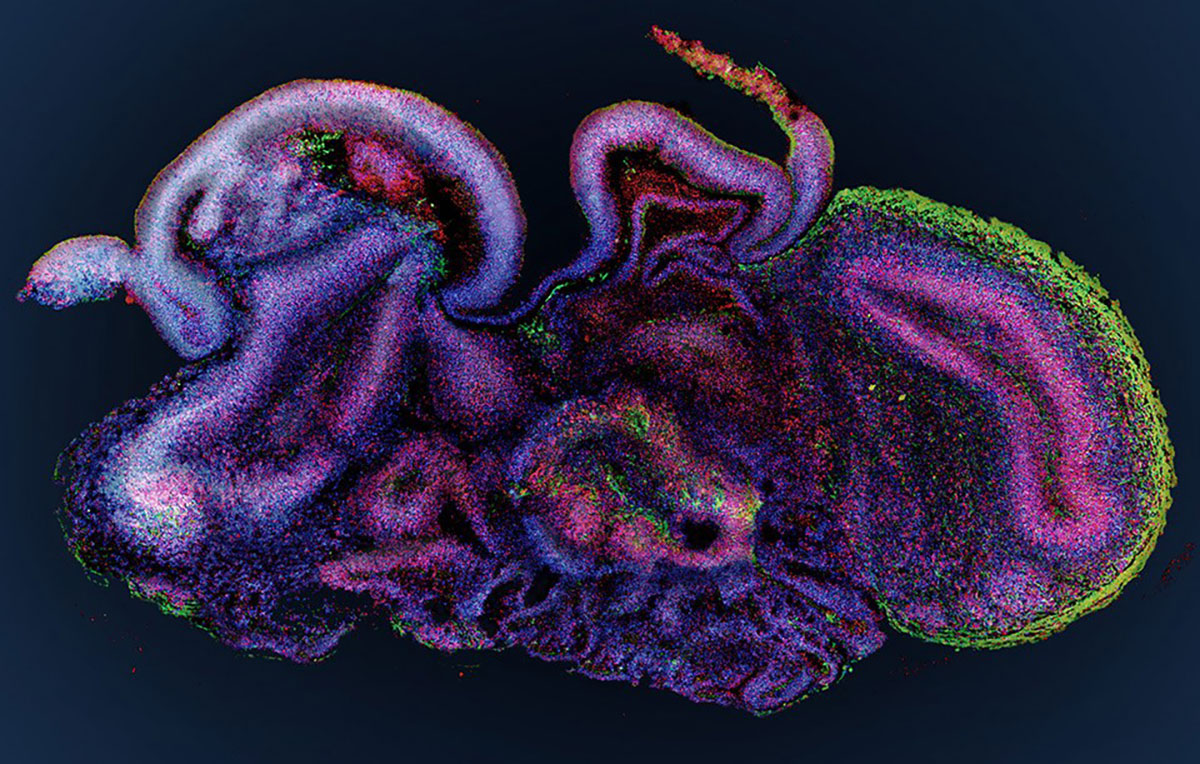
The inherent complexity barriers of the human brain necessitated brain researchers to develop more informative model systems which can recreate at least part of these complexities to study in vitro. Making use of the recently advanced protocols to create organoids from stem cells, Madeline Lancaster and colleagues were among the first to set out to study microcephaly by developing a protocol for the culture and creation of human cerebral organoids (Lancaster MA & Knoblich JA., Nature Protocols, 2014).
They discovered (similar to observations in other organoid systems) that their cerebral organoids would not only develop into spherical bundles of neuronal cells, but that various discrete, interdependent brain regions would eventually arise out of their model system.
Could these organoids recapitulate critical steps of human brain development?
To dig deeper, the authors focused on microcephaly, a developmental disorder causing arrested brain development. While there are multiple causative reasons for microcephaly, the authors focused on a single mutated causative gene, discovered by sequencing microcephaly patients. They took some skin cells from that patient, re-transformed them into pluripotent stem cells, and then used their 3D culture system to develop these patient-derived cells into cerebral organoids.

To their surprise, the patient-derived stem cells (carrying the mutation) behaved completely similar to pluripotent stem cells from healthy patients during the first days of their program. However, once they switched to neural induction media on day 6, the mutant cells would fail to develop properly. After phenotypic investigation, the researchers found out that the genetic mutation in the patient causes premature differentiation while failing to maintain progenitor cells, leading to overall smaller neural tissues, recapitulating the reduced brain size seen in microcephaly patients.
Lancaster et al. exciting work started a gold rush for neurobiologists to apply cerebral organoids as model systems to their respective research areas, and the enthusiasm is still growing. (Trujillo CA. and Muotri AR., Trends in Molecular Medicine, 2018)
A few years down the road, another groundbreaking study by Georgia Quadrato and colleagues from Harvard and MIT took up the enormous task of analyzing the gene expression of over 80,000 individual cells isolated from 31 human brain organoids. With several computational clustering and visualization techniques, the authors mapped all cells according to similarities, to identify and classify the diversity of these brain organoids’ cellular makeup.
Their comprehensive study provided for the first time a systematic overview of what many scientists already suspected; human brain organoids grow more diverse the longer they are allowed to progress. Furthermore, these bundles of increasingly diverse cells would start interacting in complicated ways, beginning to self-organize and form complex 3D structures resembling distinct brain regions. Mindblowingly, the authors also discovered that their longest-cultured (‘oldest’) brain organoids had formed light-sensitive photoreceptor cells that would give electrochemical impulses that changed spontaneous neuronal activity when stimulated with a light source. All in a dish.

The diversity and maturation of cell types generated, the robustness of the neuronal networks, the presence of structural traits of mature neurons and the possibility of using sensory experience to modulate neuronal activity collectively suggest that, beyond modeling early events of progenitor biology, these 3D brain organoids have the potential to model higher-order functions of the human brain, such as cellular interactions and neural circuit dysfunctions related to neurodevelopmental and neuropsychiatric pathologies. — Quadrato G. et al., Nature, 2017
Subsequent studies on the long-term culture of brain organoids (Gordon A., et al., Nature Neuroscience, 2021) leave no doubt that these in vitro model systems have a lot to teach us about human brain development, neuronal network dynamics, and diseases of the brain.
Based on genome-wide analysis of the epigenetic clock and transcriptomics, as well as RNA editing, we observe that three-dimensional human cortical organoids reach postnatal stages between 250 and 300 days, a timeline paralleling in vivo development. We demonstrate the presence of several known developmental milestones, including switches in the histone deacetylase complex and NMDA receptor subunits, which we confirm at the protein and physiological levels.
These results suggest that important components of an intrinsic in vivo developmental program persist in vitro. — Gordon A., et al., Nature Neuroscience, 2021
There is no question that the advent of brain organoids opened up vast new opportunities for exploration, not by ignoring the brain’s complexity barrier, but by making it more accessible.
Even more generally, we can expect that developing biotechnologies like human-derived induced pluripotent stem cells (iPSC) will offer more ways to recapitulate the complexity and functionality of human tissues and allow for the generation of novel and exciting in vitro systems including multicellular three-dimensional (3D) cultures, brain organoids, and organ-on-a-chip technologies. In short:
We might finally have a window into the inner workings of intelligent cellular networks.
However, the increasing complexity of these model systems also brings new enormous challenges for researchers and companies who want to study them. Novel methods and devices are needed to meet accuracy, precision, sensitivity, specificity, and repeatability requirements for the measurement, analysis, and evaluation of physiologically relevant endpoints in these systems, as well as biomarker discovery and screening applications. (Poli D. et al., Front. Neurosci., 2019, Costamagna G. et al., Int J Mol Sci., 2021)
Chapter 3: Towards new insights with cell-electronic interfaces
Progress in science depends on new techniques, new discoveries, and new ideas, probably in that order. — Sydney Brenner

- Electrophysiology
Electroactive tissues like the brain, heart, or nervous system are built up from networks of cells that communicate with each other via rapid depolarization of their membrane potential (see action potential or spikes) mediated by the opening and closing of voltage-gated ion channels.
Measuring this electrochemical activity with techniques like intracellular patch-clamp or extracellular electrode arrays has been challenging to say the least, and electrophysiology is among the least popular niches occupied by neuroscientists. This is understandable because the electrical activity of intelligent networks is so fickle that any interference, from magnetic fields to nearby plugged devices to even direct light (photoelectric effect) can interfere with one’s measurement. It often took a whole room full of electrical devices within a Faraday cage to correct for all the sources of electrical interference. Even more constraining was the size of electrodes, the poor spatiotemporal resolution of biosignals, and the limited ability to measure many cells simultaneously.
However, the recent miniaturization of electronics, especially semiconductor microchip technologies (CMOS), allowed researchers and engineers to tackle these problem anew with high-density multi-electrode arrays (HD-MEA) (Berdondini L. et al., IEEE International Conference on Electronics, Circuits and Systems, 2001, Imfeld K. et al., IEEE Transactions on Biomedical Engineering, 2008, Berdondini L. et al., Lab of a chip, 2009).
Since then, multi-electrode arrays have seen renewed interest, opening up the previous elusive layer of spatiotemporal detail of network dynamics from thousands of neurons (Maccione A. et al., Brain Research Bulletin, 2015 , Khambhati AN. et al., NeuroImage, 2018). And therein lies a lot of potential.
- Cell-electronic interfaces
The simplest definition of cell-electronic interfaces is that they are devices that connect biological cells to hard- or software applications.
How can microelectrode array-based cell-electronic interfaces explore new brain biology?
To answer this question, I will have to share some scientific data and results from 3Brain AG, my current employer. So please take this as an acknowledgment of my conflict of interest, a potential for bias, and consider consulting additional outside resources on the topic, technology, and its limitations.
Alright, so 3Brain AG’s cell-electronic interfaces are able to connect to thousands of cells at the same time and process biosignals at 20.000 frames per second in a spatially resolved pixel array, basically microchips not unlike the ones used in an ultra-high-speed HD camera (or CPU for that matter). This pixel approach for neuronal activity is effectively expanding the concept of label-free functional imaging beyond optical (microscopy) and optogenetic approaches and gives a unique visual representation of the mysterious language of intelligent networks by connecting them to computers in real-time (see below).
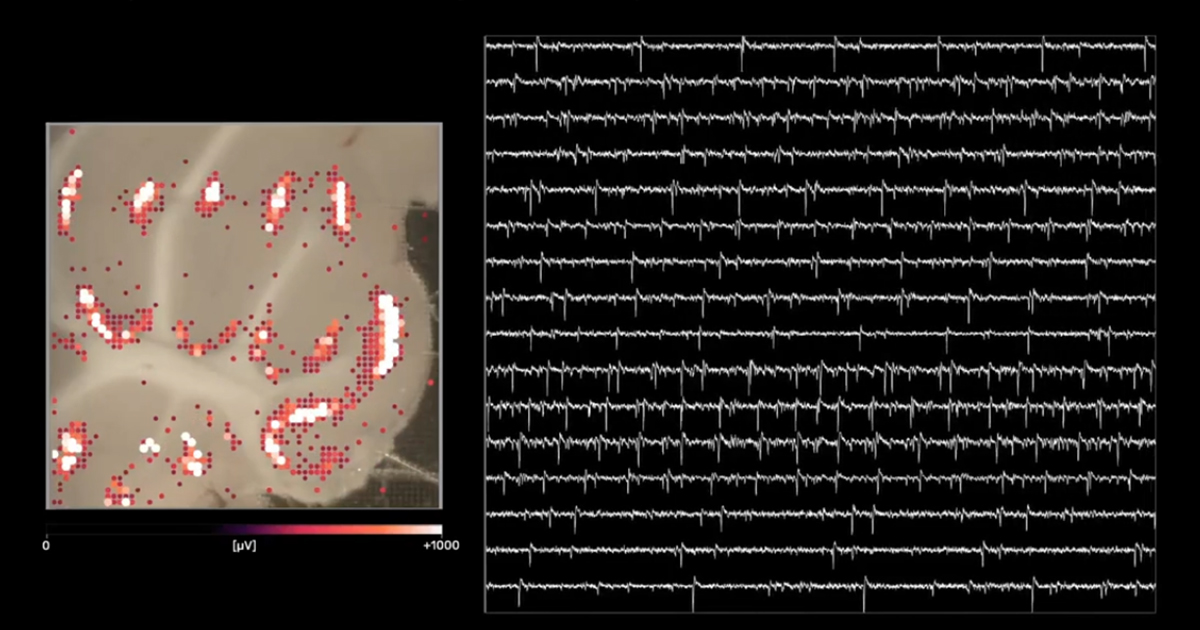
Just to explain the top image: A rat cerebellar brain slice (~200µm thick) is put on top of the electrode array. Each pixel corresponds to roughly one neuron, and from their activity, many useful parameters can be gathered, from amplitudes to firing rates to precise timing and cross-correlation with other active neurons.
Capturing all these fickle biosignals is technically challenging because measuring thousands of neurons simultaneously firing sometimes up to hundreds of times per second quickly reaches an information density that wires cannot transport, especially not in real-time without losing biosignals. Therefore, a lot of signal pre-processing, like amplification, noise filtering, and drift correction, is directly performed by the electronics on the microchip. The signals get transformed into bits and further processed by specialized hardware devices before these measured biosignals can be subsequently used in software applications where sophisticated machine-learning algorithms and statistics further process the incoming data streams to derive first and higher order metrics of neuronal network activity (more on them a bit later).
If we zoom back out again, we see that somewhere along the way, electrochemical biosignals ended up as bits to be manipulated in software.
The whole process also works bi-directionally, with software being able to direct electrical stimulations to specific pixels (and their associated neurons) of choice, causing them to depolarize.
Therefore it seems clear to me that cell-electronic interfaces like sophisticated multielectrode arrays will play an essential role in the expansion of brain biology towards information sciences.
- Measuring 3D model systems
Alright, finally we have reached the stage of the article where the puzzle pieces start falling together. We have outlined the inherent complexity of the brain, how new in vitro model systems hold high promise to recreate them, and how high-density electrode arrays can capture enormous data streams from neuronal activity.
Yet there is one major limitation left of current planar HD-MEA technologies, cell-electronic interface or not:
Planar electrodes cannot reach inside of tissues and organoids where all the interesting biology happens.
Duh. This is a problem for obvious reasons. Electrodes can only measure extracellular currents when they are in close proximity to the cells they are supposed to measure. This is feasible in planar 2D neuronal cultures (Egert U. et al., Experimental Brain research, 2002), but it does not work very well for more complex 3D model systems like brain slices or organoids (Mapelli L. et al., bioarxiv, 2022 , see comparisons below). On top of that, 3D model systems require efficient nutrient diffusion, oxygenation, and metabolic waste removal to avoid rapid necrosis of contact tissue that occurs when measuring on planar MEA surfaces (Liu L. et al., Bio Protoc., 2020).
Luckily for us, these problems are not insurmountable, as a recent pre-print describing the design, implementation, and functional validation of a novel 3D cell-electronic interface from Lisa Mapelli et al., together with 3Brain AG, shows. (again, potential conflicts of interests apply)
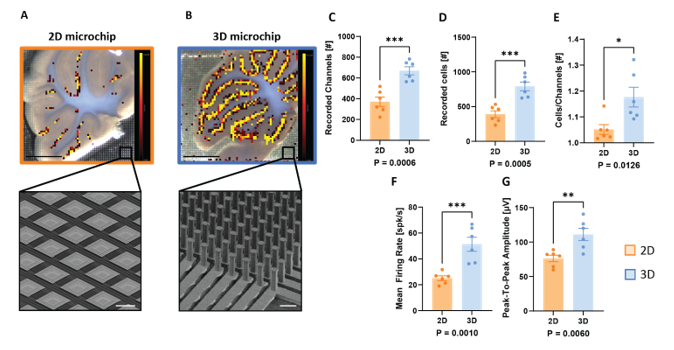
In this paper, we introduce for the first time a new fabrication technique able to provide a chip with thousands of microneedles (μneedles) with height ranging from 65 to 90 µm and width from 14 to 26 µm with 60 µm pitch. […] Notably, this fabrication process is fully CMOS-compatible, allowing the generation of the first high-density MEAs based on this technology.
The result is a monolithic CMOS high-density MEA providing a 64 by 64 μneedle electrode grid with an integrated microfluidic system to guarantee efficient oxygen, nutrient, and chemical diffusion at the bottom layers of the tissue. Such technology condenses penetrating capability, high-resolution recording, and improved tissue viability in a unique device. — Mapelli L. et al., bioarxiv, 2022
Developing a 3D cell-electronic interface technology that will enable researchers to access the complex cytoarchitecture of 3D tissues and brain organoids at unprecedented depths and granularity is something remarkable. (That is all I am going to say about this, if you want hype for marketing purposes, you can check the press release).
But let’s go back to science for a moment.
I mentioned before that currently, first and higher-order metrics can be derived from software using their connection to cellular networks, and I think it is worth looking a bit deeper into what that means.
Classical examples of these first-order metrics include canonical analyses of neuronal activity, for example, mean firing rate (how many spikes are fired in a given time frame) or Inter-Spike Intervals (time between subsequent action potentials), or the amplitude and duration of a local field potential.
Higher-order metrics such as Spike correlation analysis (which evaluates how spike firing patterns between two neurons are correlated, which is indicative of a functional connection between them) or Field potential bursts (LFPs that repeat in a pattern over time interspersed by periods of quiescence) will be essential tools in functional connectomics, network and graph-theoretic approaches, as well as hint at new biological discoveries, for example how distinct neuronal networks with different spiking patterns and network activity can arise within the same brain organoid (unpublished, see below)
These results are encouraging the belief that machine-learning-based software solutions will be critical to understanding meta-patterns of the formation and language of these intelligent biological networks.

In summary, cell-electronic interfaces produce a new class of information and metrics that can be used to identify and evaluate the phenotypes of biological preparations and provide a functional readout in for example perturbation experiments, neurotoxicity-, or compound screening approaches.
Lastly, a uniquely interesting aspect of these advanced cell-electronic interfaces is that they transform the data depths of intelligent biological networks into information that can be navigated with software and algorithms, including for example pattern recognition by intelligent computational networks.
This fruitful symmetry might lead to advancements in the understanding of both computational and biological intelligence.
(But that is certainly a topic for another day)
Conclusion
In this (overly long, sorry!) blog post, we outlined some of the major limitations that have plagued brain research for a long time, and how technological innovations, from stem cells to new culture techniques all the way to intelligent microchip design and AI software have reached a critical threshold of sophistication to finally tackle some of the biggest questions in the field.
Why, where, and how does spontaneous activity emerge in brain organoids and how do coordinated brain waves start? Does neuronal activity shape cytoarchitecture or is it the other way round? How does connectivity evolve over time? Are there differences in neuronal development in organoids derived from healthy versus at-risk patients, and can we treat them? Can we discover novel drug classes that modulate neuronal activity and shape brain architecture?
For many, collecting or exchanging cellular information directly from the inside of tissues and organoids through hardware connected to a computer might sound like science fiction, but it has become a science fact. The next challenge will be making sense of the massive data streams these novel model systems and interface technologies offer scientists to explore.
No matter how one looks at it, it will be an exciting time for scientific inquiry and human ingenuity. Maybe we will finally break some of the complexity barriers of the brain, maybe not. One thing is however certain:
Cell-electronic interfaces are a technology here to stay.
A small, hopeful afterword
About 15 years ago, 3Brain AG (or better said, some of the researchers who would eventually co-found the company) developed the first high-definition microelectrode pixel array based on complementary metal oxide semiconductor (CMOS) chip technology.

These microchips are commonly used for deep tech applications like high-definition digital cameras or CPUs for computation. While passive electrode arrays and methods like patch clamps existed before, 3Brain’s advanced 2D cell-electronic interfaces allowed researchers to observe intelligent networks at a scale and precision previously unseen.
For the first time, scientists could get a peek at the bigger picture of intelligent networks, and what we learned is that there is beauty to be found in the complexity of the unknown.

The development of 3D microchips with thousands of thin microneedles which can penetrate the cytoarchitecture of brain organoids and tissues while retaining tissue integrity, together on-chip processing, amplification, and noise filtering of biosignals marks another remarkable leap in technological progress. It is an interesting time to work in this space, but for the interested reader, one question still remains in the room:
So… Will we ever be able to fully understand the complexities of the human brain?
To be honest, the jury is still out. Skepticism is a healthy attribute to have for scientists in the weeds of the everyday struggles and limitations of research. The complexity barriers of the brain are real and will likely remain insurmountable for a long time, so I think acknowledging that is okay. All too often, we expect the scientific process to be the motor for innovation, to build new shiny gadgets for our convenience, or find better treatments for diseases that ail us.
What we tend to forget sometimes is to appreciate that science is also an engine of hope.
In talking to many scientists and engineers over the years, it couldn’t be clearer to me that we are all explorers at heart. The human brain might or might not be the most complex structure in the known universe, but there is little doubt that its self-organizing dynamic 3D cytoarchitecture is a galaxy inside of us… in wait for exploration.
Recent years saw an unprecedented effort to recreate that 3D galaxy in vitro, through the development of model systems like brain organoids, or by getting ever more precise insights into the inner life of brain slices or preparations. Novel cell-electronic interfaces provide a unique window of opportunity to study these fascinating in vitro systems, make new discoveries and test new ideas.
Progress in science depends on new techniques, new discoveries, and new ideas, probably in that order. — Sydney Brenner
In the larger scale of scientific inquiry, connecting to intelligent networks with software marks an expansion from cell chemistry to network complexity, from brain biology to information technology, and from passively listening to having an active conversation. This is beyond exciting.
For too long, we had to rely on magnifying glasses and maps, simulations, and incomplete metaphors when it comes to the brain. Now, we finally have some tools to dare venture into that last great unknown.
We don’t know yet what lies beyond that horizon, but if I had to wager a bet today, my money would be that what we find on this journey will be hauntingly beautiful.
And the fact we finally can take steps to find out sounds quite hopeful to me.
References
Berdondini L. et al., IEEE International Conference on Electronics, Circuits and Systems, 2001
Egert U. et al., Experimental Brain research, 2002
Imfeld K. et al., IEEE Transactions on Biomedical Engineering, 2008
Berdondini L. et al., Lab of a chip, 2009
Bullmore, E. and Sporns, O., Nat. Rev. Neurosci., 2009
Shephard GM., Front. Neuroanat., 2011
Lancaster MA et al., Nature, 2013
Lancaster MA & Knoblich JA., Nature Protocols, 2014
Fornita A. et al., Nat Rev Neurosci, 2015
Maccione A. et al., Brain Research Bulletin, 2015
Katrin Amunts K and Zilles K, Neuron, 2015
Voss P. et al., Frontiers in Psychology, 2017
Quadrato G. et al., Nature, 2017
Khambhati AN. et al., NeuroImage, 2018
Savtchouk I. and Volterra A, JNeurosci, 2018
Trujillo CA. and Muotri AR., Trends in Molecular Medicine, 2018
Poli D. et al., Front. Neurosci., 2019
Bassi MS. et al., Int. J. Mol. Sci., 2019
Wojtasik M et al., Front. Neuroanat., 2020
Wu Q. et al., BioMedical Engineering Online, 2020
Amuts K. et al., Science, 2020
Interactive multilevel human brain atlas, Human Brain Project
BRAIN Initiative Cell Census Network (BICCN), Nature, 2021
Jiang C. et al., bioarxiv, 2021
Costamagna G. et al., Int J Mol Sci., 2021
Gordon A., et al., Nature Neuroscience, 2021
Wu YK. and Zenke F., eLife, 2021
Mapelli L. et al, bioarxiv, 2022
Copyright:
Featured articles may include proprietary company information on products or research. For educational and other non-commercial purposes, you are allowed to share (copy and redistribute in any medium or format) & adapt (remix, transform, and build upon) the material as long as you give proper attribution (see also: CC BY-NC 3.0 license)
Declaration of conflict of interest:
The author is an employee at 3Brain and the article contains proprietary information and product mentions.
Acknowledgments:
I want to thank Lisa Mapelli and colleagues from the University of Pavia in Italy for sharing real-time recordings and analysis of various brain slices with Accura-3D (3Brain AG, Switzerland) ahead of publication. I also would like to thank a:head bio AG for sharing some organoid measurements performed with Accura-3D.